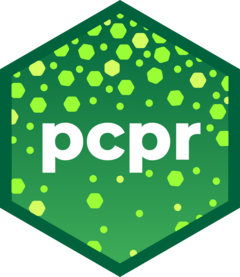
Retrieve default PCP parameter settings for given matrix
Source:R/get_pcp_defaults.R
get_pcp_defaults.Rd
get_pcp_defaults()
calculates "default" PCP parameter settings lambda
,
mu
(used in root_pcp()
), and eta
(used in rrmc()
) for a given data
matrix D
.
The "default" values of lambda
and mu
offer theoretical guarantees
of optimal estimation performance. Candès et al. (2011) obtained the
guarantee for lambda
, while
Zhang et al. (2021)
obtained the result for mu
. It has not yet been proven whether or
not eta
enjoys similar properties.
In practice it is common to find different optimal parameter values
after tuning these parameters in a grid search. Therefore, it is
recommended to use these defaults primarily to help define a reasonable
initial parameter search space to pass into grid_search_cv()
.
Value
A list containing:
lambda
: The theoretically optimallambda
value used inroot_pcp()
.mu
: The theoretically optimalmu
value used inroot_pcp()
.eta
: The defaulteta
value used inrrmc()
.
The intuition behind PCP parameters
root_pcp()
's objective function is given by:
$$\min_{L, S} ||L||_* + \lambda ||S||_1 + \mu ||L + S - D||_F$$
lambda
controls the sparsity ofroot_pcp()
's outputS
matrix; larger values oflambda
penalize non-zero entries inS
more stringently, driving the recovery of sparserS
matrices. Therefore, if you a priori expect few outlying events in your model, you might expect a grid search to recover relatively largerlambda
values, and vice-versa.mu
adjustsroot_pcp()
's sensitivity to noise; larger values ofmu
penalize errors between the predicted model and the observed data (i.e. noise), more severely. Environmental data subject to higher noise levels therefore require aroot_pcp()
model equipped with smallermu
values (since higher noise means a greater discrepancy between the observed mixture and the true underlying low-rank and sparse model). In virtually noise-free settings (e.g. simulations), larger values ofmu
would be appropriate.
rrmc()
's objective function is given by:
$$\min_{L, S} I_{rank(L) \leq r} + \eta ||S||_0 + ||L + S - D||_F^2$$
eta
controls the sparsity ofrrmc()
's outputS
matrix, just aslambda
does forroot_pcp()
. Because there are no other parameters scaling the noise term,eta
can be thought of as a ratio betweenroot_pcp()
'slambda
andmu
: Larger values ofeta
will place a greater emphasis on penalizing the non-zero entries inS
over penalizing the errors between the predicted and observed data (the dense noiseZ
).
The calculation of the "default" PCP parameters
lambda
is calculated as \(\lambda = 1 / \sqrt{\max(n, p)},\) where \(n\) and \(p\) are the dimensions of the input matrix \(D_{n \times p}\) Candès et al. (2011).mu
is calculated as \(\mu = \sqrt{\frac{\min(n, p)}{2}},\) where \(n\) and \(p\) are as above [Zhang et al. (2021)].eta
is simply \(\eta = \frac{\lambda}{\mu}\).
References
Candès, Emmanuel J., Xiaodong Li, Yi Ma, and John Wright. "Robust principal component analysis?." Journal of the ACM (JACM) 58, no. 3 (2011): 1-37.
Zhang, Junhui, Jingkai Yan, and John Wright. "Square root principal component pursuit: tuning-free noisy robust matrix recovery." Advances in Neural Information Processing Systems 34 (2021): 29464-29475. [available here]
Examples
# Examine the queens PM2.5 data
queens
#> # A tibble: 2,443 × 27
#> Date Al NH4 As Ba Br Cd Ca Cl
#> <date> <dbl> <dbl> <dbl> <dbl> <dbl> <dbl> <dbl> <dbl>
#> 1 2001-04-04 NA 1.62 NA NA NA NA NA NA
#> 2 2001-04-07 0 2.66 0 0.012 0.00488 0 0.0401 0.0079
#> 3 2001-04-13 0.0094 1.41 0.0016 0.024 0.00211 0.004 0.036 0
#> 4 2001-04-19 0.0104 1.22 0.001 0.006 0.00422 0 0.0543 0.003
#> 5 2001-04-25 0.0172 0.723 0.0024 0.015 0.00117 0 0.0398 0
#> 6 2001-05-01 0.0384 3.48 0.0017 0.041 0.00873 0.001 0.136 0
#> 7 2001-05-04 0.0964 6.22 0.0025 0.039 0.0111 0 0.137 0
#> 8 2001-05-07 0.004 0.233 0.001 0.016 0.00263 0 0.055 0.0054
#> 9 2001-05-10 0.0547 2.04 0.001 0.055 0.00521 0 0.121 0.001
#> 10 2001-05-13 0.0215 0.229 0 0.021 0.00122 0 0.0249 0
#> # ℹ 2,433 more rows
#> # ℹ 18 more variables: Cr <dbl>, Cu <dbl>, EC <dbl>, Fe <dbl>, Pb <dbl>,
#> # Mg <dbl>, Mn <dbl>, Ni <dbl>, OC <dbl>, K <dbl>, Se <dbl>, Si <dbl>,
#> # Na <dbl>, S <dbl>, Ti <dbl>, NO3 <dbl>, V <dbl>, Zn <dbl>
# Get rid of the Date column
D <- as.matrix(queens[, 2:ncol(queens)])
# Get default PCP parameters
default_params <- get_pcp_defaults(D)
# Use default parameters to define parameter search space
scaling_factors <- sort(c(10^seq(-2, 4, 1), 2 * 10^seq(-2, 4, 1)))
etas_to_grid_search <- default_params$eta * scaling_factors
etas_to_grid_search
#> [1] 5.611340e-05 1.122268e-04 5.611340e-04 1.122268e-03 5.611340e-03
#> [6] 1.122268e-02 5.611340e-02 1.122268e-01 5.611340e-01 1.122268e+00
#> [11] 5.611340e+00 1.122268e+01 5.611340e+01 1.122268e+02